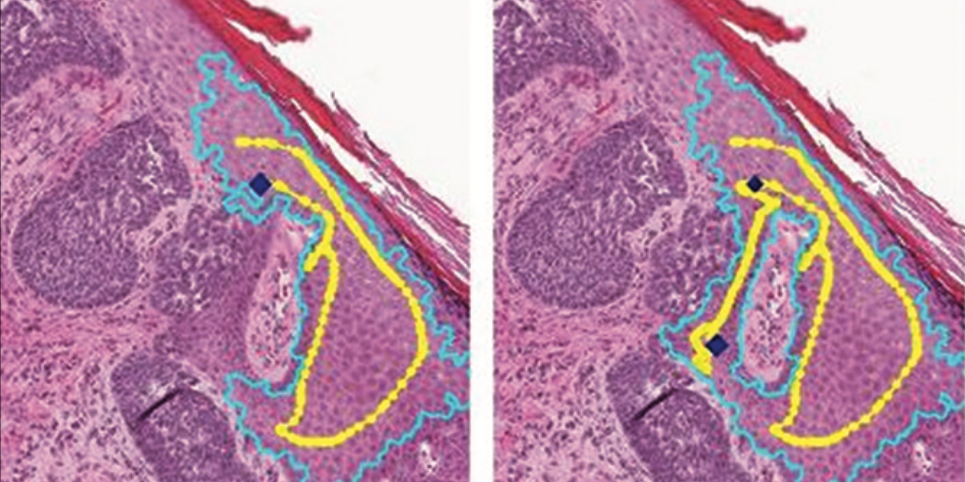
Abstract
Background: Recent advancements in machine learning (ML) bring great possibilities for the development of tools to assist with diagnostic tasks within histopathology. However, these approaches typically require a large amount of ground truth training data in the form of image annotations made by human experts. As such annotation work is a very time-consuming task, there is a great need for tools that can assist in this process, saving time while not sacrificing annotation quality. Methods: In an iterative design process, we developed TissueWand – an interactive tool designed for efficient annotation of gigapixel-sized histopathological images, not being constrained to a predefined annotation task. Results: Several findings regarding appropriate interaction concepts were made, where a key design component was semi-automation based on rapid interaction feedback in a local region. In a user study, the resulting tool was shown to cause substantial speed-up compared to manual work while maintaining quality. Conclusions: The TissueWand tool shows promise to replace manual methods for early stages of dataset curation where no task-specific ML model yet exists to aid the effort.
Citation
Martin Lindvall,
Alexander Sanner,
Fredrik Petré,
Karin Lindman,
Darren Treanor,
Claes Lundström,
TissueWand, a rapid histopathology annotation tool
Journal of Pathology Informatics, 11(1): 27, doi:10.4103/jpi.jpi_5_20, 2020.
Acknowledgements
We would like to thank pathologist Gordan Maras for providing valuable input on the usability of the tool when applied to lymph node metastases. The study was partially supported by Visual Sweden and by the Wallenberg AI, Autonomous Systems and Software Program (WASP). Martin Lindvall and Claes Lundström are employed by Sectra AB.